Chair: Xiaofei Wang, PhD (Duke)
Instructors:
Shu Yang, PhD (NCSU)
Mingyang Shan, PhD (Eli Lilly)
Xiaofei Wang, PhD (Duke)
Course Description:
External controls (ECs) from historical randomized clinical trials (RCTs) or real-world data (RWD) can be used to construct or augment the comparator arm to support regulatory and healthcare decision-making. This has seen increasing use in pediatrics, rare diseases, and diseases with high unmet needs. However, heterogeneity between the RCT and RWD stemming from covariate shift, posterior drift, or unmeasured confounding can lead to biased treatment effect estimates and incorrect decision-making. To enable robust decision-making, careful considerations need to be given to the design and analysis of EC trials to mitigate potential biases. This course will provide an overview of the statistical framework and challenges in EC trials, robust Bayesian and Frequentist methods to adaptively borrow ECs using data similarity metrics to mitigate biases due to data heterogeneity, developments in sensitivity analysis to evaluate the robustness of ‘no unmeasured confounding’ and other untestable assumptions in EC settings, and considerations in the power analysis and sample size determination for EC designs. Simulated case studies and R code will be integrated throughout the course to accompany the methodology and concepts presented. A basic understanding of clinical trials, GLM, hypothesis testing, survival analysis, and Bayesian statistics will be helpful for this course.
We propose to cover the following topics in our half-day short course:
- Overview of Hybrid Controls, Bias + Heterogeneity Concerns: We will review key statistical issues for hybrid controlled trials in a causal inference framework focusing on treatment effect estimand, identification assumptions, and the impact on method validity and robustness due to measured and unmeasured confounders.
- Bayesian Adaptive Borrowing Methods: We will discuss Bayesian adaptive methods to borrow from external control data including 1) overview of common informative priors (power, commensurate, mixture, meta-analytic predictive, and variations), 2) data-driven methods to construct prior distributions using similarity metrics (propensity score, prior predictive p-values, Bayes factors), and 3) evaluation of operating characteristics under different bias scenarios.
- Frequentist / Semi-parametric Analysis Methods : We will discuss Frequentist methods for mitigating biases from external controls, including calibration, weighting, doubly robust approaches to address covariate incomparability, test-then-pool, selective borrowing, and bias function modeling to handle outcome incomparability.
- Sensitivity Analysis: We will present recent developments to conduct sensitivity analysis for unmeasured confounding and other untestable causal identifiability assumptions using both Bayesian and Frequentist borrowing approaches.
- Prospective Design of Hybrid Controlled Trials: To tie theory and methodology with practical application, we will review key considerations when designing a hybrid-controlled trial. Sample size determination and power analysis will be explored using simulation under different assumptions about the comparability of covariates shift and outcomes shift between concurrent controls (CCs) and external controls (ECs).
• Case Studies with Interactive Programming: Case studies and R scripts will be provided to illustrate the approaches with additional discussion on statistical inference and type I error control.
Instructors:
Shu Yang, PhD
Associate Professor
Department of Statistics
North Carolina State University
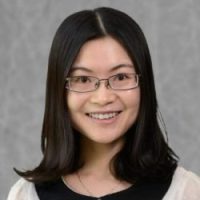
Shu Yang (PhD from Iowa State University in Mathematics and Statistics) is an Associate Professor of Statistics in the Department of Statistics at North Carolina State University. Her primary research interest is causal inference and data integration, particularly with applications to comparative effectiveness research in health studies. She also works extensively on methods for missing data and spatial statistics. She has been Principal Investigator for several U.S. NSF, NIH, and FDA research projects.
Mingyang Shan, PhD
Director, Real World and Access Analytics
Eli Lilly and Company
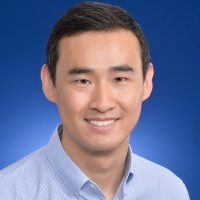
Mingyang Shan (PhD in Biostatistics from Brown University) is a director in Real World and Access Analytics at Eli Lilly and Company. His research interests include causal inference, tokenization & record linkage, and data integration in clinical trial design, including designs incorporating external controls. He is a major collaborator on an FDA U01 grant on using RWE for regulatory decision-making and is a member of the ASA Biopharmaceutical section RWE and AI/ML working groups.
Xiaofei Wang, PhD
Professor
Department of Biostatistics & Bioinformatics
Duke University School of Medicine
Director of Statistics
Alliance Statistics and Data Center
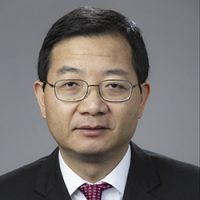
Xiaofei Wang (PhD in Biostatistics from the University of North Carolina, Chapel Hill) is a Professor of Biostatistics and Bioinformatics at Duke University School of Medicine and the Director of Statistics for Alliance Statistics and Data Center. He has extensive experience supporting cancer clinical trials for Alliance/CALGB and Duke Cancer Institute. NIH and FDA have funded his methodology research focusing on adaptive clinical trials, statistical methods for predictive and diagnostic medicine, and integrative methods for analyzing patient data from multiple sources. He is an elected fellow of the American Statistical Association (ASA).