Chair: Herb Pang, PhD (Genentech)
Vice Chair: Chuan Hong, PhD (Duke)
Instructors:
Paul Schuette, PhD (FDA)
Chris Harbron, PhD (Roche)
Yong Chen, PhD (UPenn)
Course Description:
In this short course, we will have three instructors coming from FDA, pharma industry, and academia.
The FDA instructor will focus on selected FDA AI/ML Discussion Papers and Guidance Documents. FDA has published multiple discussion papers and guidance documents on artificial intelligence and machine learning (AI/ML). This session will discuss these topics along with Good Machine Learning Practices (GMLP). A focus will be on considerations for the use of AI/ML in the FDA regulated drug development process.
The industry instructor will focus on the challenges for developing predictive models. With the increasing availability of data at scale, the pharmaceutical industry is looking to generate predictive models for a wide variety of applications to support the development and utilisation of its products. We will discuss some of the challenges and potential solutions associated with working with large scale data to develop predictive models that are trustworthy and likely to be adopted, including quantifying uncertainty, modelling across diseases and data modalities, ensuring the fairness and interpretability of our models and dealing with structured missing data. The academic instructor will focus on the use of GPTs in RWE generation. Generative pre-trained transformers (GPTs) have transformed natural language processing but lack focus on generating causal evidence from Real-World Data (RWD). We present RWE-GPT, the first GPT model tailored for RWD, incorporating negative control outcomes (NCOs) for debiasing. Pretrained on large datasets and fine-tuned on smaller, relevant cohorts, RWE-GPT uses an adversarial objective to address unmeasured confounders. Evaluated on data from over 20 million patients, it demonstrates robust performance in causal estimation tasks, supporting applications in drug repositioning and counterfactual modeling, showcasing the potential of foundation models in advancing real-world evidence generation.
Instructors:
Paul Schuette, Ph.D.
Deputy Division Director
Division of Analytics and Informatics
Office of Biostatistics, Center for Drug Evaluation and Research (CDER)
U.S. Food and Drug Administration (FDA)
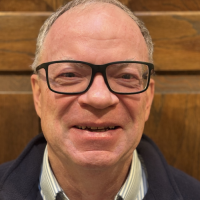
Paul Schuette is the Deputy Division Director for the Division of Analytics and Informatics in FDA’s Office of Biostatistics. Paul joined the FDA in 2008, after previously working in academia and as a government contractor. Paul supervises a team of statistical analysts who support the statistical and scientific programming needs of the Office of Biostatistics. Additionally, Paul is a CDER representative on FDA’s Scientific Computing Board and serves as a representative on CDER AI council and other FDA AI/ML working groups. Paul received his M.A. and Ph.D. from the University of Wisconsin-Madison, and his B.S. degree in mathematics from Kansas State University.
Chris Harbron, Ph.D.
Expert Statistical Scientist
Methods, Collaboration and Outreach (MCO), Data Sciences
Roche Pharmaceuticals

Chris Harbron is an Expert Statistician leading capabilities in Advanced Analytics within the Data Sciences function at Roche. Through a variety of roles within the pharmaceutical industry Chris has worked in all stages of the drug development pipeline from drug discovery to early and late development. In recent years Chris has focussed on the development of ML/AI and how it can be most impactful used within the pharmaceutical industry including leading external collaborations such as with the Alan Turing Institute.
Yong Chen, Ph.D.
Professor, Biostatistics
Director, Center for Health AI and Synthesis of Evidence (CHASE)
University of Pennsylvania
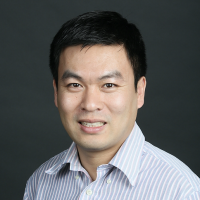
Yong Chen is a Professor of Biostatistics and Founding Director of the Center for Health AI and Synthesis of Evidence (CHASE) at the University of Pennsylvania, where he leads research in clinical evidence generation and synthesis using real-world data. He also directs the Penn Computing, Inference, and Learning (PennCIL) lab, focusing on developing methods for integrating clinical data.
Dr. Chen is one of twenty Commissioners serving internationally on the Lancet Commission on Rare Diseases. He is also a Statistical Editor for the Annals of Internal Medicine, a Statistical Consultant for New England Journal of Medicine-AI, and an Associate Editor for both the Journal of the American Statistical Association – Applications and Case Studies (JASA-ACS) and The Annals of Applied Statistics (AoAS).
Dr. Chen has authored over 200 peer-reviewed papers in statistics and medical informatics, with ongoing research support from the NIH, AHRQ, and PCORI. His work centers at evidence synthesis, machine learning/AI, and clinical evidence generation. He is an elected Fellow of the American Statistical Association and the American College of Medical Informatics, with joint appointments in Applied Mathematics and at the Penn Institute for Biomedical Informatics.