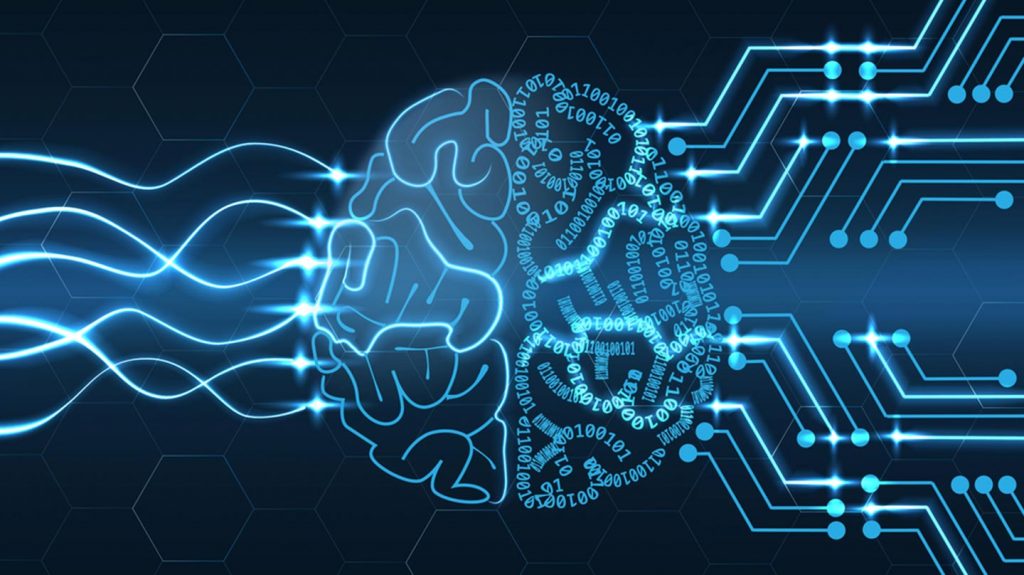
About the course
This course explores the interdisciplinary conversation between economics and artificial intelligence (AI). In experiential education, this course shows how the two disciplines advance each other by an explainable AI approach: economics makes AI more explainable by clarifying causal relationship and AI empowers economic applications by increasing efficiency. Advanced research in Microeconomics, Macroeconomics, and Behavioral and Experimental Economics is covered with both a general literature review and a case study. The course concludes with a research proposal where students propose an executive plan for academic research and automated products collaboratively in a team of Economist, Data Scientist, and Data Engineer.
Following the DKU 7 animating principles of collaborative problem-solving, research and practice, lucid communication, collaborative problem-solving, rooted globalism, purposeful life, and wise leadership, in this course, you would prosper together as DKUers, accomplish productive takeaways for your profiles, and exercise leadership as a global citizen.
Learning Objectives
Bloom’s Taxonomy: remember, understand, apply, analyze, evaluate, create
By the end of this course, you will be able to:
1. Explain, in both definition and real-world cases, the concept of explainable AI and how it empowers jointly the research and practice in Economics and Data Science. (remember and understand)
2. Illustrate in three hands-on technologies, interdisciplinary big data, behavioral experiments, quantitative computing, how Explainable AI can be applied to solve economic and social issues with both causal inference and predictive efficiency. (understand, apply, and analyze)
3. Elaborate a data science pipeline and divisions of labors in a research team of Economist, Data Scientist, Data Engineer, and how each role contributes to an Explainable AI research. (apply, analyze, evaluate, create)
4. Initiate a promising proposal of research with an explainable AI approach through 1) understanding the contribution of existing literature from 5 facets: backgrounds and motivations, research questions, research methodologies, research results, intellectual merits, and practical impacts. 2) identifying the limitations of existing literature and proposing a promising research question; 3) drafting an executive plan including the data science pipeline and the division of labors (4) communicating the research proposal effectively (5); learning collaboratively in providing constructive critics to peers’ research proposal and making revisions responding to critics (apply, analyze, evaluate, create)